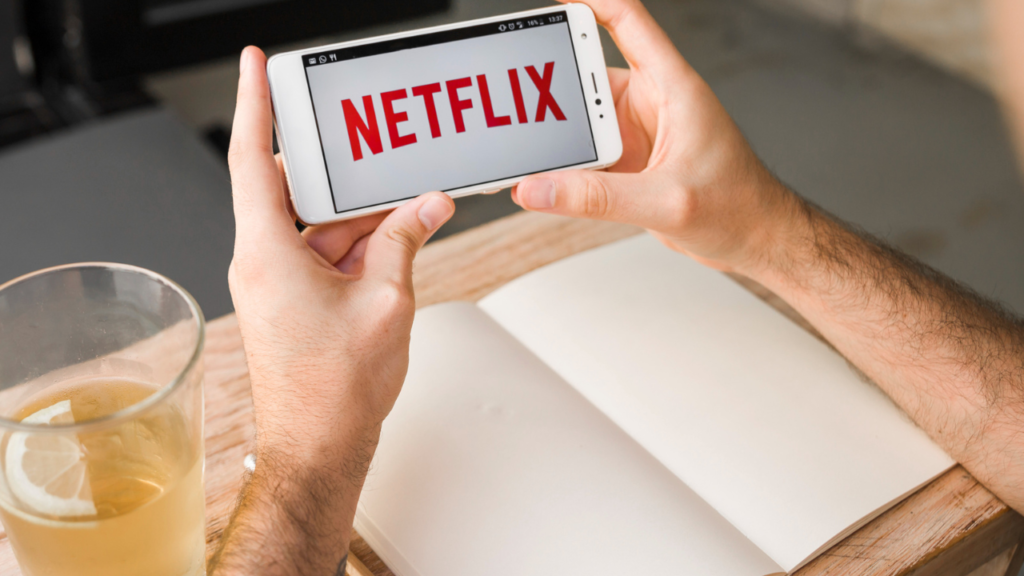
Netflix Recommendations: How Netflix Uses AI
In the world of on-demand entertainment, Netflix stands as a pioneer. With millions of subscribers worldwide, Netflix is more than a streaming service – it’s an advanced technology company with strong AI, data science and machine learning (ML) capabilities. But how does Netflix leverage these technologies to personalize the viewer experience and make recommendations to keep users hooked? Let’s discuss how Netflix uses AI, data science, and ML to fuel its recommendation engine and create the perfect viewing experience.
Table of Contents
1. The Power of Personalization
Netflix’s strength lies in its ability to offer highly personalized recommendations, whether for TV shows, movies or documentaries. When you open the platform, it doesn’t just present a random list of trending titles — each suggestion is tailored to your personal preferences. AI plays a central role here, helping Netflix deliver customized content to more than 230 million subscribers worldwide.
Each Netflix profile is essentially treated as a separate data entity. Over time, Netflix collects a large amount of data, such as:
- Genres you see frequently.
- The time of day you like to watch.
- The devices you use.
- You want or like to watch single episodes.
Using this data, Netflix’s algorithms can continuously learn more about your preferences, allowing the platform to create a personalized experience.
2. Collaborative Filtering: The Backbone of Recommendations
Netflix’s recommendation system relies heavily on collaborative filtering, a data-driven technique widely used in the field of data science. Collaborative filtering works by analyzing a user’s past behavior — such as viewing history, ratings and interactions — and comparing it to the behavior of other similar users.
For example, if User A and User B have the same viewing history, then User A has watched a new show, Netflix can suggest that show to User B, predicting that they will like it based on taste similarity.
In addition to user-based collaborative filtering, Netflix also uses item-based collaborative filtering. This approach recommends content based on relationships between different shows or movies, taking into account viewing behavior patterns across its entire user base.
3. Content-Based Filtering: Deep Learning in Action
In addition to collaborative filtering, Netflix uses content-based filtering to recommend titles. Here, machine learning and deep learning techniques analyze the content metadata of each show or movie. This metadata includes genre, cast, plot keywords, director, and even audio and video quality.
Por ejemplo, si eres fanático de los programas de ciencia ficción con un determinado conjunto de actores o temas argumentales, los algoritmos de Netflix identificarán estos elementos y recomendarán otros programas con características similares. A través del aprendizaje profundo, la plataforma puede descubrir relaciones ocultas dentro del contenido, sugiriendo a menudo títulos que no habrían sido evidentes con los métodos de filtrado tradicionales.
4. Natural Language Processing (NLP) for Tagging and Metadata
Natural Language Processing (NLP) is another important tool that Netflix uses to analyze text-based information, such as show descriptions and user reviews. This helps create a broader understanding of each content beyond genre labels, allowing for more nuanced recommendations.
Netflix has an internal system called AVA (Automatic Video Annotation), which uses NLP and computer vision to break down videos and extract detailed metadata. AVA helps create highly specific tags, such as “strong female leads” or “mind-bending sci-fi,” that help refine recommendations.
5. The Role of Reinforcement Learning
One of Netflix’s most exciting uses of AI is its application of reinforcement learning. Reinforcement learning allows algorithms to learn from user actions in real time, improving recommendations with every click, scroll and viewing decision.
For example, Netflix experiments with thumbnail and show placement. When users interact with a particular thumbnail or trailer, the algorithm learns which design or presentation is more effective. Over time, this fine-tuning leads to more interesting recommendations.
Netflix facilitates A/B testing to optimize every user-facing element, from which titles to how the platform’s interface should be designed. These tests help Netflix understand which combinations lead to more satisfied users.
6. Optimizing the Viewing Experience with Data Science
Beyond recommendations, Netflix uses data science to improve other areas of the user experience. For example:
- Streaming Quality: Netflix applies data science to optimize video delivery, ensuring smooth streaming even on slow internet connections. The algorithm dynamically adjusts resolution based on network conditions.
- Content Production: Netflix uses data insights to decide which shows or movies to produce or license. By analyzing global viewership patterns, Netflix can identify potential content that will resonate with large audiences or niche segments.
7. Tackling the “Cold Start” Problem
One of the biggest challenges facing recommendation systems is the “cold start” problem – how to recommend content to new users with no prior history. To overcome this, Netflix primarily relies on general data based on demographic information, such as location, age, and popular titles in your area. It encourages new users to select their preferred genre or specific title during the onboarding process.
Once the system collects more user-specific data, such as what you’ve seen and liked, the recommendations become more accurate and personalized.
8. AI in Content Thumbnails and Trailers
Even Netflix’s visuals are powered by AI and ML Netflix’s algorithms analyze which thumbnails, artwork and trailers perform well for different users. For example, the platform might display a more comedic thumbnail for a rom-com if you prefer lighter content, while showing a more dramatic image for someone who favors intense drama. This hyper-targeted visual marketing is another way Netflix personalizes the viewing experience, making it more interesting and engaging.
Conclusion
Netflix’s success is a testament to the power of AI, data science and machine learning in the entertainment industry. How the platform uses technology to meet and fulfill the needs of its users is just one aspect of its sophisticated recommendation system. By leveraging collaborative filtering, deep learning, natural language processing, and reinforcement learning, Netflix creates an ever-evolving, personalized experience for every customer. And as AI technology continues to advance, the future of Netflix’s recommendations will only get smarter, keeping viewers coming back for more.